Knowledge Discovery in Immunological Cell Types
Precision Immunology is a means to better understand how (and in whom) immunotherapy drugs work. These therapies act on subsets of immune cells, and the relative abundance of particular cell types before, during, or after treatment may predict patient outcomes. The associations between immune cell subsets and disease states are often established using technologies that deeply profile the immune system.
The Challenge
New technologies, such as high parameter flow cytometry and single cell RNA sequencing, can interrogate the immune system with unprecedented depth, and are often employed in Precision Immunology studies. These technologies measure a myriad of genes and proteins at once, and the combinations of these molecules define distinct cell types, traits, and functions. Precision Immunology studies often reveal cell subsets for which there exists very little information.
According to Pratip K. Chattopadhyay, who directs a Precision Immunology Laboratory, interpretation of data from new technologies is currently an enormous challenge. Researchers in his lab have spent countless hours searching through PubMed or Google Scholar for information connected to the cell subsets that they discover.
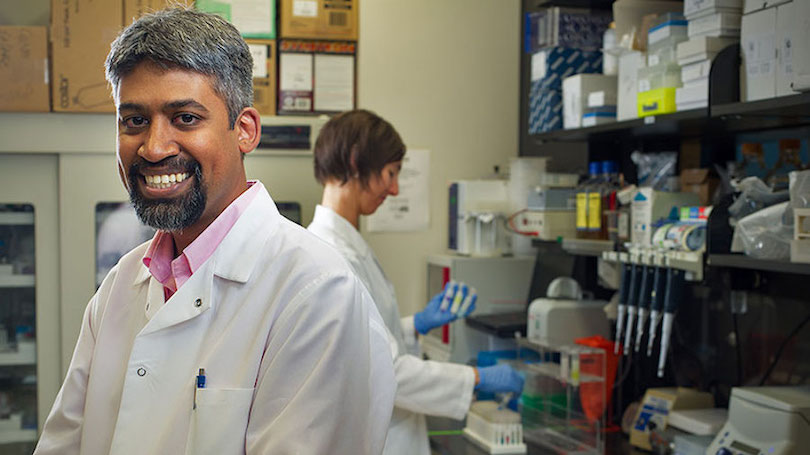
Dr. Chattopadhyay explained, “we often found interesting adjacent leads, such as a disease, a pathway, or a clinical trial, but it was difficult to follow-up such leads. Each lead required extensive new search criteria, and those results were often outside the context of the original query.”
With so many potential leads, the lab found it increasingly difficult to make connections across the information they obtain. “We felt like there was no way to organize the information we were obtaining, and we were sure that we were missing things. In sum, it’s been difficult to gain insight from our results using common approaches,” Dr. Chattopadhyay noted.
Solution: From Cell Type to Insight
Dr. Chattopadhyay met with Epistemic AI to discuss the information challenges faced by researchers in his lab. A pilot study was performed to test whether the Epistemic AI platform was suitable for knowledge discovery in immunology, and whether it provided more information than tools already in use.
“The results were remarkable,” Dr. Chattopadhyay noted. Using the Epistemic AI platform, he and his team could easily search for and cross reference proteins and diseases of interest, and reveal the publications, clinical trials, and pathways most relevant to their work. “We could then quickly review this information and update our queries to expand or re-focus our understanding. The platform ranked results to fit our interests, so that we didn’t waste time wading through irrelevant information,” he added. “Best of all, we developed new leads about the cell types we were interested in,” he concluded.
Following this pilot, Dr. Chattopadhyay’s team used the Epistemic AI platform to gain further insight into results that were previously published in a study of melanoma patients (JCI Insight). Dr. Chattopadhyay’s group found that CD38+ CD39+ T-cells were expanded in certain patients who didn’t respond to immunotherapy. After creating a custom knowledge map around these T-cells with the Epistemic AI platform, investigators quickly learned:
- both CD38 and CD39 are part of an adenosinergic pathway that suppresses central memory T-cell proliferation in the tumor microenvironment,
- providing a mechanistic hypothesis for diminished anti-tumor responses and poor survival in patients with more of these cells,
- and suggesting that systemic immunity (and COVID vaccine efficacy) might be affected in these people.
The Epistemic AI platform also identified an inhibitor of this pathway that might be a more effective part of immunotherapy regimens in these patients.
Methodology: the Knowledge Map in Action
The remainder of this white paper takes a deeper look at how Epistemic AI’s platform helped the Chattopadhyay lab learn more about a potentially important cell type in melanoma immunotherapy.
In 2020, Dr. Chattopadhyay’s group used 28-color flow cytometry to study melanoma patients who were treated with different sequences of two immunotherapy drugs: anti-PD1 and anti-CTLA4. The goal of the study was to identify cell types whose frequency was associated with the survival differences originally observed between patient groups. In addition, the study sought to identify a candidate biomarker for immunotherapy response. The study revealed an interesting type of T-cell, which expressed both CD38 and CD39, that was elevated in patients with poor survival. The results of the study were published in JCI Insight in 2020, but the paper didn’t discuss the function of these cells, mainly because the authors did not know much about them (despite extensive searches in known sources such as PubMed and Google Scholar). Thus, the objective for using the Epistemic AI platform was to try and understand more about the function of the CD38+ and CD39+ cells, which was impossible to achieve with conventional search techniques.
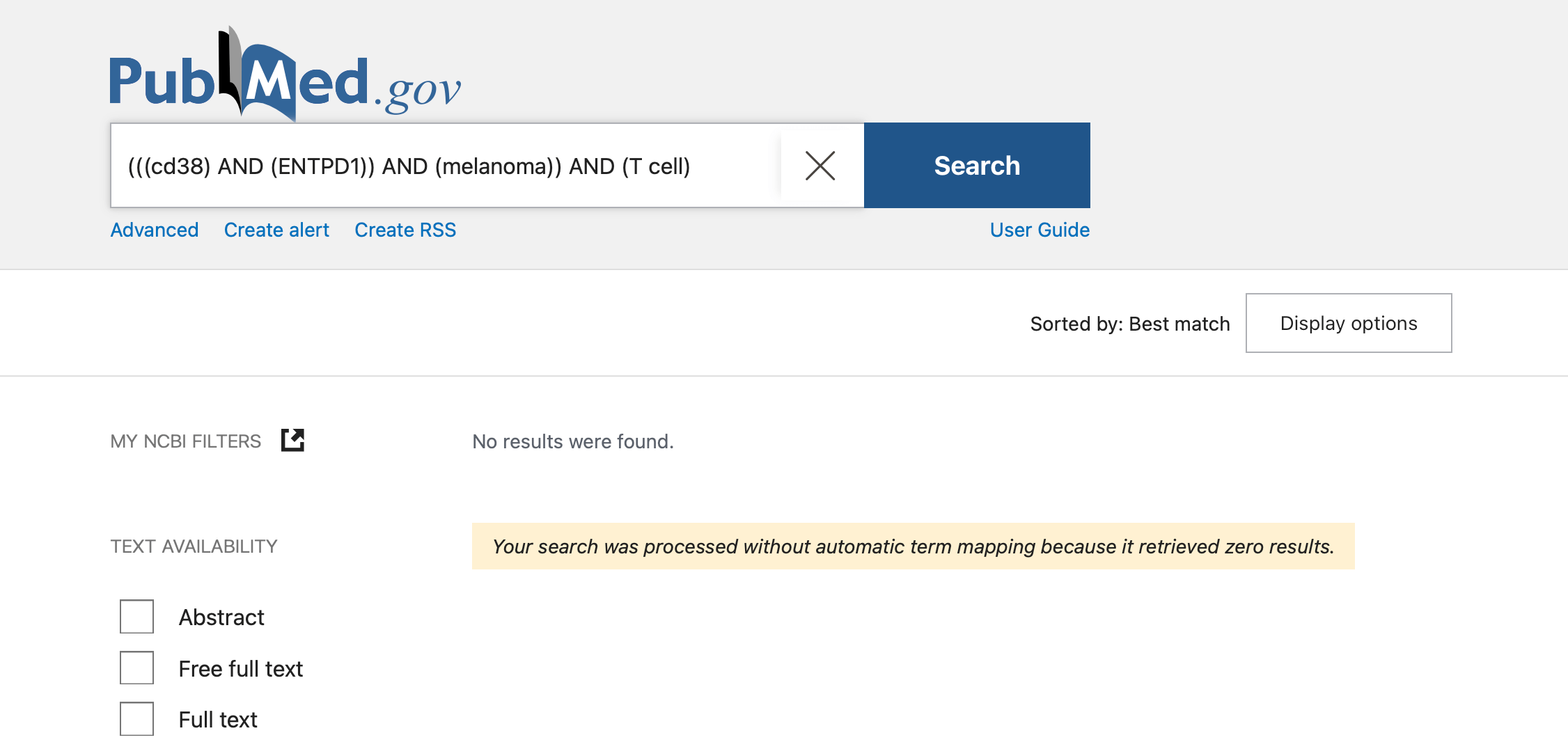
With the Epistemic AI platform, the Chattopadhyay Lab began building a Knowledge Map by entering the human genes CD38 and CD39 (recognized by the Epistemic AI platform as an alias for ENTPD1), the disease melanoma and the cell type “T-Cell.” While there are many search engines that can provide a search for these “keywords” the unique AI research assistant provided an auto-filled approach that allowed Dr. Chattopadhyay to quickly form the query, and the Knowledge Map rapidly found all of the relationships among the queried entities.
Within seconds after selecting the entities and sending the query, the Knowledge Map presented a kaleidoscope of results that were related to the query. In particular, it was readily apparent that there is a shared function of the molecules found in hydrolyzing ATP and ADP (red arrows), which was hitherto unknown to Dr. Chattopadhyay.
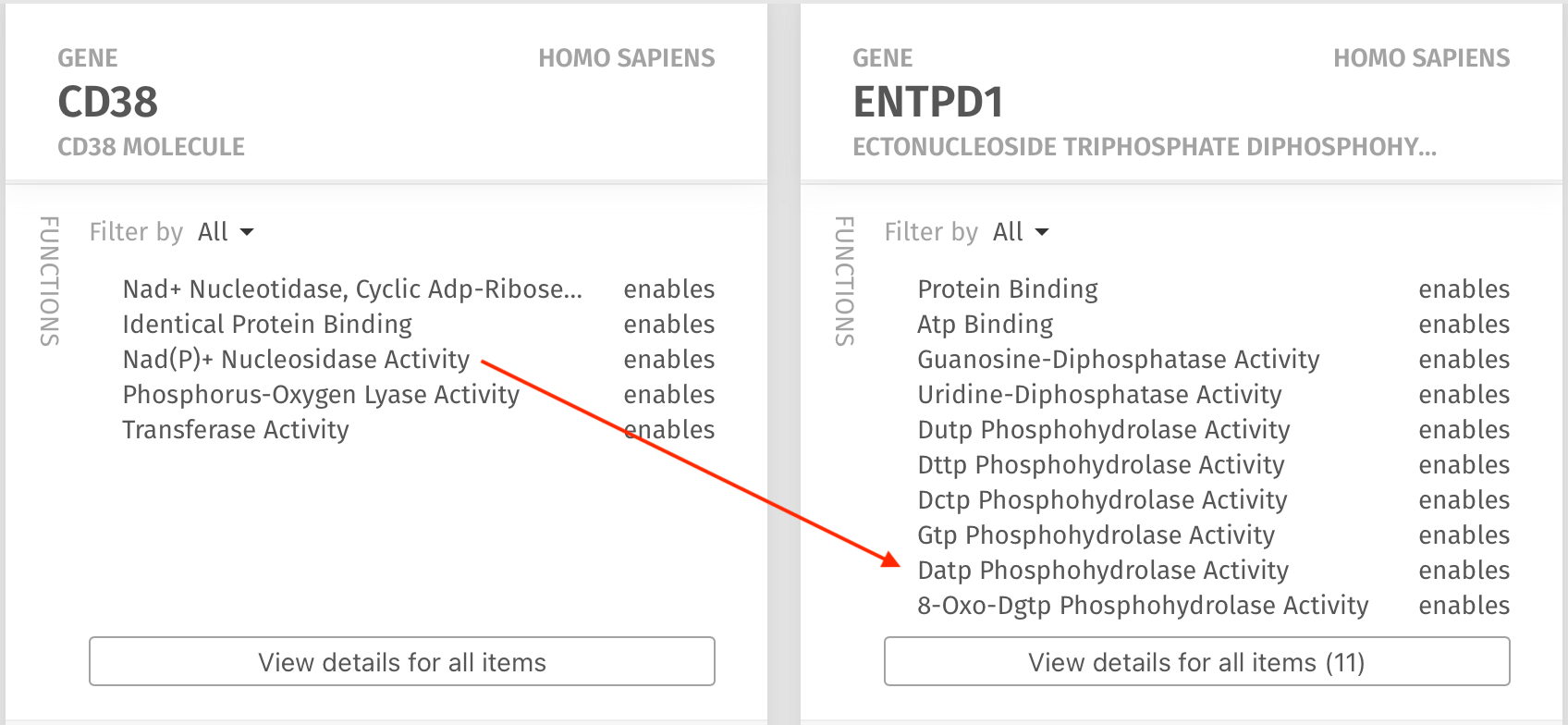
Next, the Chattopadhyay lab wanted to know what existing drugs, if any, target CD38 and CD39, and which might help to develop an hypothesis for therapies that can target these potentially pathogenic cells. This required no effort as the Knowledge Map already provided an ordered ranking of drugs known to interact with the molecules: for CD38 were two antibody drugs - Isatuximab and daratumumab - and for CD39, a compound that appeared in the Chembl database of drug screens.
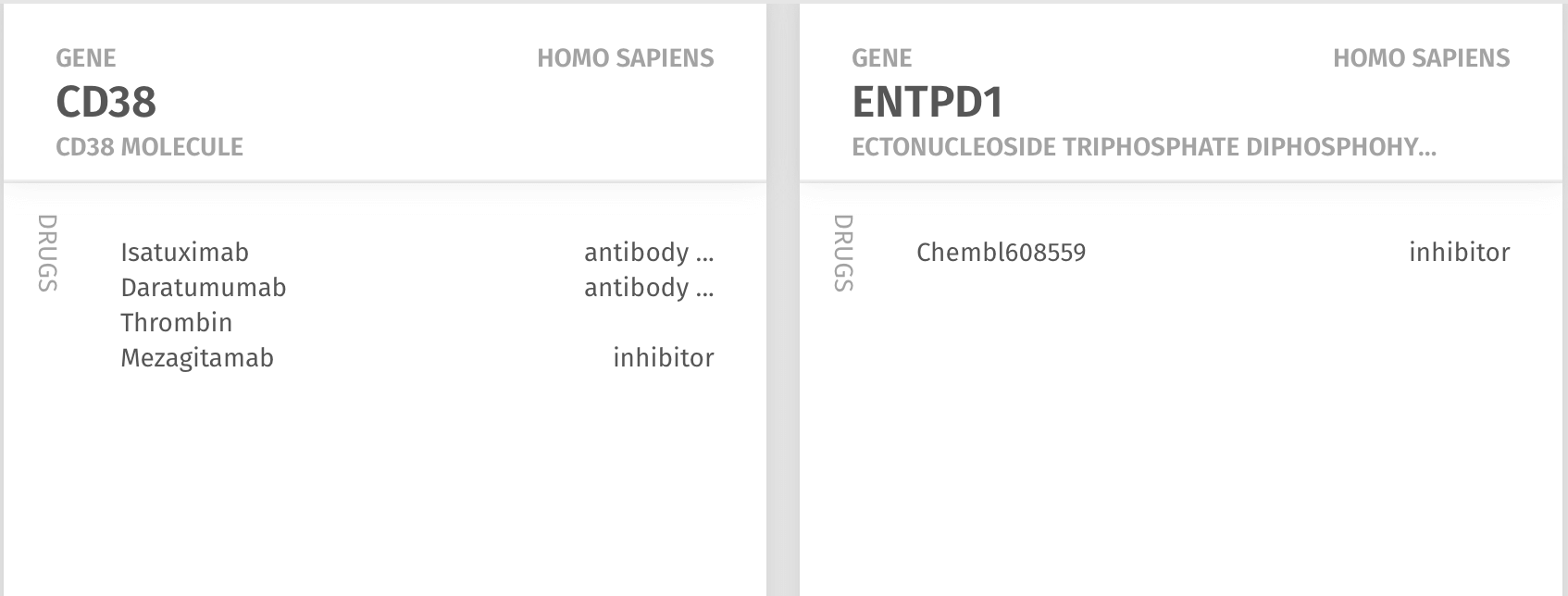
Following from this, certain biological processes were also presented by the Knowledge Map, and a paper was quickly discovered that noted CD38 is involved in apoptotic signaling and NAD metabolism (which was also indicated in the biological functions).
The discovery process described above took only a few minutes, during which Dr. Chattopadhyay could develop a mechanistic hypothesis involving metabolism and cell suicide. From these few insights, Dr. Chattopadhyay set out to fully explore the performance of the Knowledge Map, and specifically to see what information could be specifically found on the relationship among these molecules, in melanoma, as expressed by T-cells.
With additional refinement of the knowledge map query, using filters, Dr. Chattopadhyay found one single paper that discussed a relationship among CD38, CD39 and melanoma. Interestingly, these molecules were studied on melanoma cells, not T-cells, giving rise to two key insights that helped to confirm part of the initial hypothesis:
- that there were no prior mentions in the literature of a connection between CD38+ CD39+ T-cells in melanoma, which indicated this was a novel finding, and
- the same molecules - CD38 and CD39 - on melanoma cells create an immunosuppressive environment that paralyzes T-cells
In this example, the majority of references were derived from the genes queried. However, the Knowledge Map offers many different perspectives for the same query. With continued exploration, examining connections from the perspective of melanoma surfaced additional clues about CD38 and CD39 expression on T-cells that fit like perfect pieces in a puzzle.
One article described an incredible network of cell surface molecules and metabolites that shape the immune response at sites of inflammation (Rivas-Yáñez et al., Int J Mol Sci, 2020). The article painted a vivid picture of how immune responses in tumors can be suppressed. Aside from the immune exhaustion molecules PD1 and CTLA4, which are common immunotherapy targets, there are regulatory T-cells in tumors that inhibit immune responses. These regulatory cells are commonly defined by their expression of FoxP3 and CD25 (as well as CD127); however, when regulatory T-cells express CD38 and CD39, they become resistant to the high levels of ATP present in a tumor, and are more potent and long-lived immunosuppressors.
Within an hour on the platform, Dr. Chattopadhyay created a Knowledge Map that built upon an experimental observation (i.e., melanoma patients fare poorly if they have high levels of CD38+ CD39+ T-cells). He was able to “quickly recite the function of the molecules, the drugs that are available to target them, what pathways and processes the molecules invoke, and whether our finding was unique.” Perhaps most importantly, Dr. Chattopadhyay found that melanoma cells could evade the immune response by expressing CD38 and CD39 themselves, and that the CD38+ CD39+ cells he found were particularly potent at immunosuppression because they had an unusual survival capacity in the hostile ATP-rich tumor microenvironment. Thus, for the patients who did poorly, high levels of CD38+ CD39+ cells might reflect more immunosuppression and consequently a reduced ability to kill off the tumor.
Conclusions
By using the Epistemic AI platform, researchers in Dr. Chattopadhyay’s Precision Immunology Laboratory were able to significantly shorten their research path from information to insight.
In the melanoma study, Epistemic AI helped to quickly define a potential function for cell type that was hitherto unknown, and even identified a drug that might target this functional pathway.
In comparison with search engines that rely upon ineffective “keyword” searches, or platforms that deliver stochastic results based on inflexible AI rules, the Epistemic AI platform provides an innovative heuristic approach to knowledge discovery where conclusions focus on the user enquiry and objectives.